Clustering/KMeansExample
Machine Learning
Clustering KMeans
The Example:
Clustering/KMeansExample
Description
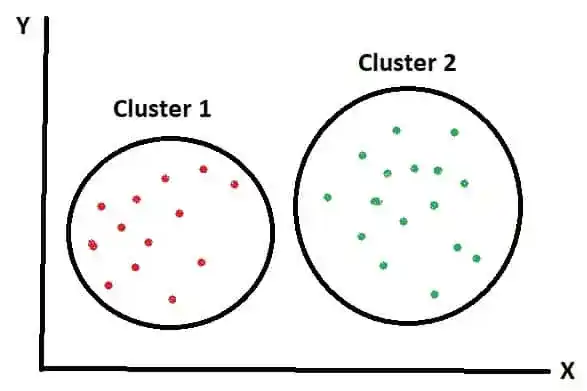
Clustering
Flink ML is a library which provides machine learning (ML) APIs and infrastructures that simplify the building of ML pipelines. Users can implement ML algorithms with the standard ML APIs and further use these infrastructures to build ML pipelines for both training and inference jobs.
Prerequisites
- JDK 11
- Maven 3.9.9
- Flink 1.17.0
KMeansExample.java
/*
* Licensed to the Apache Software Foundation (ASF) under one
* or more contributor license agreements. See the NOTICE file
* distributed with this work for additional information
* regarding copyright ownership. The ASF licenses this file
* to you under the Apache License, Version 2.0 (the
* "License"); you may not use this file except in compliance
* with the License. You may obtain a copy of the License at
*
* http://www.apache.org/licenses/LICENSE-2.0
*
* Unless required by applicable law or agreed to in writing, software
* distributed under the License is distributed on an "AS IS" BASIS,
* WITHOUT WARRANTIES OR CONDITIONS OF ANY KIND, either express or implied.
* See the License for the specific language governing permissions and
* limitations under the License.
*/
package org.apache.flink.ml.examples.clustering;
import org.apache.flink.ml.clustering.kmeans.KMeans;
import org.apache.flink.ml.clustering.kmeans.KMeansModel;
import org.apache.flink.ml.linalg.DenseVector;
import org.apache.flink.ml.linalg.Vectors;
import org.apache.flink.streaming.api.datastream.DataStream;
import org.apache.flink.streaming.api.environment.StreamExecutionEnvironment;
import org.apache.flink.table.api.Table;
import org.apache.flink.table.api.bridge.java.StreamTableEnvironment;
import org.apache.flink.types.Row;
import org.apache.flink.util.CloseableIterator;
/** Simple program that trains a KMeans model and uses it for clustering. */
public class KMeansExample {
public static void main(String[] args) {
StreamExecutionEnvironment env = StreamExecutionEnvironment.getExecutionEnvironment();
StreamTableEnvironment tEnv = StreamTableEnvironment.create(env);
// Generates input data.
DataStream<DenseVector> inputStream =
env.fromElements(
Vectors.dense(0.0, 0.0),
Vectors.dense(0.0, 0.3),
Vectors.dense(0.3, 0.0),
Vectors.dense(9.0, 0.0),
Vectors.dense(9.0, 0.6),
Vectors.dense(9.6, 0.0));
Table inputTable = tEnv.fromDataStream(inputStream).as("features");
// Creates a K-means object and initializes its parameters.
KMeans kmeans = new KMeans().setK(2).setSeed(1L);
// Trains the K-means Model.
KMeansModel kmeansModel = kmeans.fit(inputTable);
// Uses the K-means Model for predictions.
Table outputTable = kmeansModel.transform(inputTable)[0];
// Extracts and displays the results.
for (CloseableIterator<Row> it = outputTable.execute().collect(); it.hasNext(); ) {
Row row = it.next();
DenseVector features = (DenseVector) row.getField(kmeans.getFeaturesCol());
int clusterId = (Integer) row.getField(kmeans.getPredictionCol());
System.out.printf("Features: %s \tCluster ID: %s\n", features, clusterId);
}
}
}
Run
Build the Examples
# https://github.com/apache/flink-ml
mvn clean package -DskipTests
Copy
flink-ml-examples-1.17-2.4-SNAPSHOT.jar
flink-ml-uber-1.17-2.4-SNAPSHOT.jar
statefun-flink-core-3.2.0.jar
to
flink-1.17.0/lib.
Start local cluster (Flink 1.17.0):
./bin/start-cluster.sh
Run
KMeansExample:
./bin/flink run -c org.apache.flink.ml.examples.clustering.KMeansExample ./lib/flink-ml-uber-1.17-2.4-SNAPSHOT.jar ./lib/statefun-flink-core-3.2.0.jar ./lib/flink-ml-examples-1.17-2.4-SNAPSHOT.jar
Open
http://localhost:8081
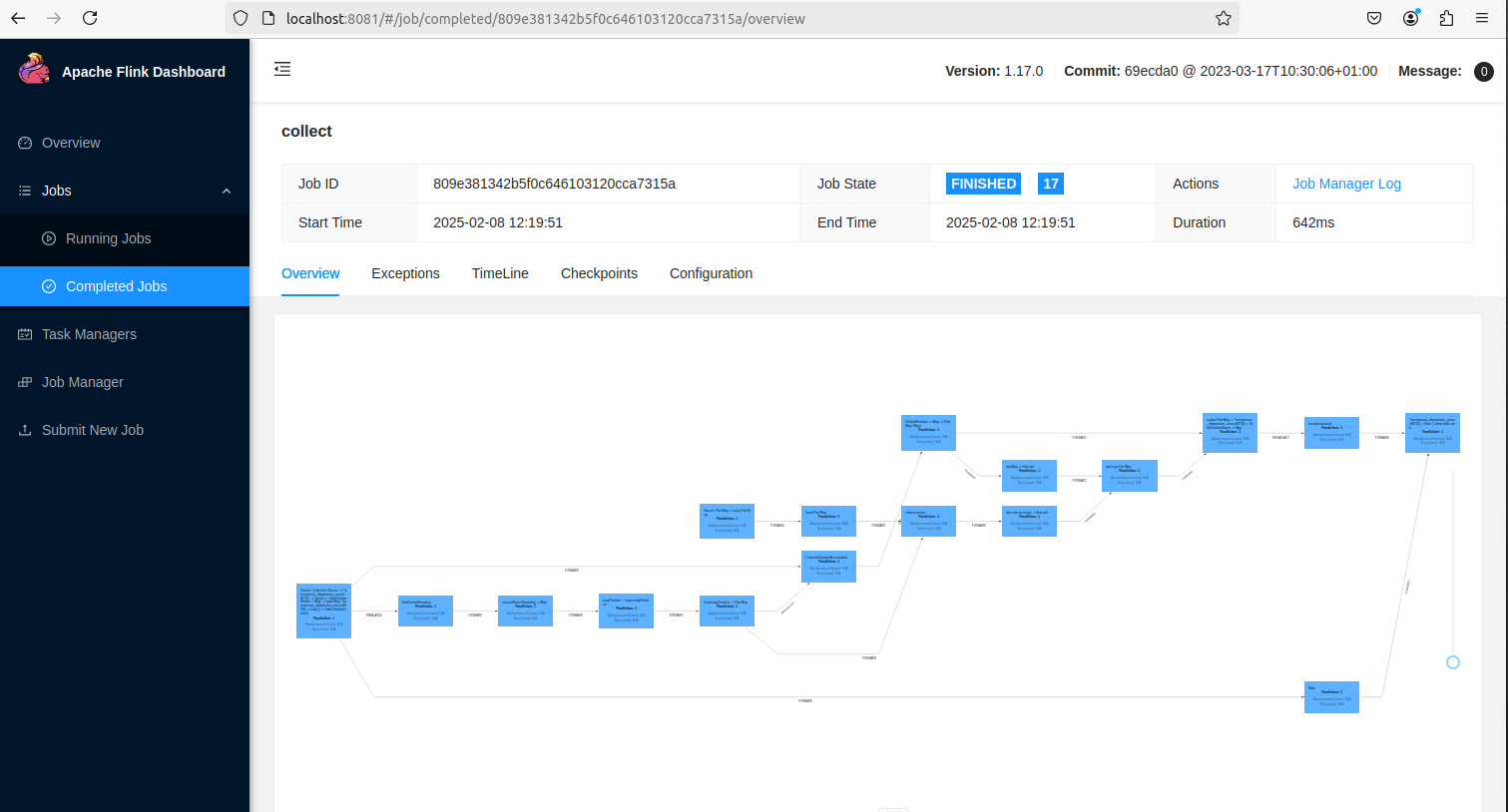
Output
Features: [0.0, 0.0] Cluster ID: 1
Features: [0.0, 0.3] Cluster ID: 1
Features: [0.3, 0.0] Cluster ID: 1
Features: [9.0, 0.0] Cluster ID: 0
Features: [9.0, 0.6] Cluster ID: 0
Features: [9.6, 0.0] Cluster ID: 0